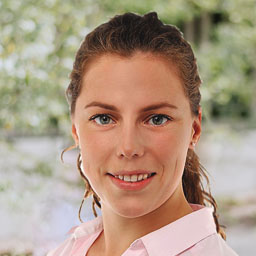
Von Kirsten Kleim
21 Juni 2021A brief review:
In Article 1, it became clear that AI can develop very impressive capabilities in response to well-defined tasks. What is artificial intelligence? | Banian AG
Using Analytical Process Automation (APA) software - "Alteryx", a neural network (an algorithm in AI) was trained and implemented in Article 2 so that it can subsequently be easily used by business users. What is artificial intelligence? | Banian AG
In this application in Article 3, it became clear that AI, and especially neural networks, are really only good at things that have been learned in training. However, if there is data that has not been learned in training, neural networks can make very unpredictable errors. And even errors that we as humans would not necessarily consider logical, because the neural network does not analyze data the way we humans do. What ist artificial intelligence? - Part 3 | Banian AG
So, all in all, neural networks are very impressive tools if they are trained and applied correctly! Tools that can also generate interesting business benefits for SMEs. - But how? In this article, we will illustrate in an implementation process flow how exactly such an AI project could be implemented.
How do I get customized AI for my business? - The implementation process flow
From a company's perspective, an AI project can go through 3 phases:
1. initial interview
In an initial meeting, the focus is on core questions. What is to be achieved? What data is available? Can AI also achieve the desired goal with this data? This is mainly about moving the desired question into the realm of possibility.
2. puncture
First of all, this is about data. Where do they come from? In what context are they? Is there perhaps other data needed? Are they sufficiently clean? Are solutions (labels) available or do they still need to be generated? Once the data is available, it is then a matter of training an initial model. With this preliminary result, a puncture pipeline is then developed. At the completion of this step, an exemplary model is ready to make preliminary predictions.
3 Operationalization
In this third section, the preliminary cut-through model is fine-tuned. In this process, model parameters are optimized, but also the data basis can be improved, for example by adding more data or better cleaning existing data. When the pipeline development is completed, the pipeline is connected to the enterprise system, tested and finally handed over.
This last phase takes the longest, and to ensure that the AI solution is truly tailored, regular consultations and introductions with the company are important.
From the perspective of a data scientist, an AI pipeline has further components, some of which are run through several times. The actual data science part, in which a neural network is trained, for example, is comparatively small. In contrast, the amount of time required to merge data and process it should not be underestimated.
For operationalization, the cycle of data preparation and model training is repeated several times to develop the optimal pipeline and get the best out of the data. Different models are developed and trained and their performance compared with each other to identify the most promising model architecture. Once the final pipeline with the best model has been identified, the customized AI solution can then be connected to the enterprise systems at the appropriate point. Depending on the application, it may be useful to implement monitoring that tracks the performance of the model and pipeline so that timely intervention can be taken if, for example, the data basis has changed.
Why a customized AI solution?
As highlighted in Article 3, AI solutions, like neural networks, are tools with impressive capabilities - but only under two conditions: They are trained according to the problem at hand, and they are applied according to their capabilities to avoid unpredictable errors. Therefore, it is essential to tailor the data science pipeline as precisely as possible to the business requirement. In a customized AI solution, the model development is already very specific to the peculiarities of the business data. The capabilities and limitations of the model based on the existing training data are thus well known and can be clearly communicated. In addition, the connection of the model is also customized. This ensures that the model is applied according to its capabilities.
Any ideas yet?
Are there any process steps that might also fit into the field of AI? Or is there a forecast that has actually always been interesting? But is this really the right use case for AI?
We at Banian AG are happy to help with an initial consultation. Contact us!
Addendum:
Or would a few more use cases help? In the next article, we will discuss where you could use AI as an SME based on a few examples.